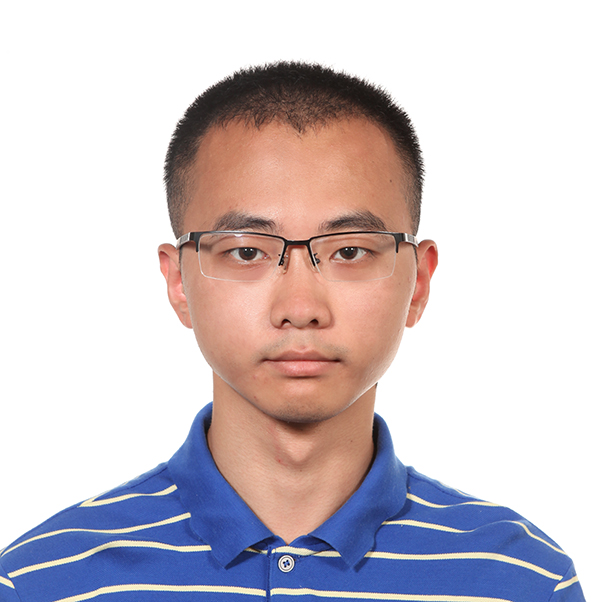
Han Cai
hcai.hm [at] gmail (dot) com
I am a research scientist at NVIDIA Research. Before joining NVIDIA, I received my doctoral degree at MIT EECS. I received my master's and bachelor's degrees at Shanghai Jiao Tong University (SJTU).
My research interests lie in artificial intelligence and machine learning, particularly efficient foundation models (diffusion models, LLMs, etc), EdgeAI and AutoML.
Email / Google Scholar / GitHub / Twitter / Linkedin
Awards
Competition Awards
- Third Place in the 2022 ACM/IEEE TinyML Design Contest at ICCAD
- First Place in the 2020 IEEE Low-Power Computer Vision Challenge, CPU detection & FPGA track
- First Place in the 2019 IEEE Low-Power Image Recognition Challenge, classification & detection track
- First Place in the Low-Power Computer Vision Workshop at ICCV 2019, DSP track
- Third Place in the CVPR 2019 Low-Power Image Recognition Challenge, classification track
News
- Mar 26 2025: PS3 is accepted by CVPR 2025.
- Jan 22 2025: DC-AE, SANA, COAT, and HART are accepted by ICLR 2025.
- Apr 06 2024: EfficientViT-SAM is accepted by eLVM@CVPR'24.
- Feb 29 2024: CAN and DistriFusion are accepted by CVPR 2024.
- Sep 12 2023: EfficientViT is highlighted by MIT home page and MIT News.
- July 18 2023: EfficientViT: Multi-Scale Linear Attention for High-Resolution Dense Prediction is accepted by ICCV 2023.
- Mar 2022: Lite Pose: Efficient Architecture Design for 2D Human Pose Estimation is accepted by CVPR'2022.
- Jan 2022: Network Augmentation for Tiny Deep Learning is accepted by ICLR'2022.
- Sep 2020: TinyTL: Reduce Activations, Not Trainable Parameters for Efficient On-Device Learning is accepted by NeurIPS'2020.
- Feb 2020: APQ: Joint Search for Network Architecture, Pruning and Quantization Policy is accepted by CVPR'2020.
- Dec 2019: Once-For-All Network (OFA) is accepted by ICLR'2020.
- Dec 2018: ProxylessNAS: Direct Neural Architecture Search on Target Task and Hardware is accepted by ICLR'2019.
Selected Projects
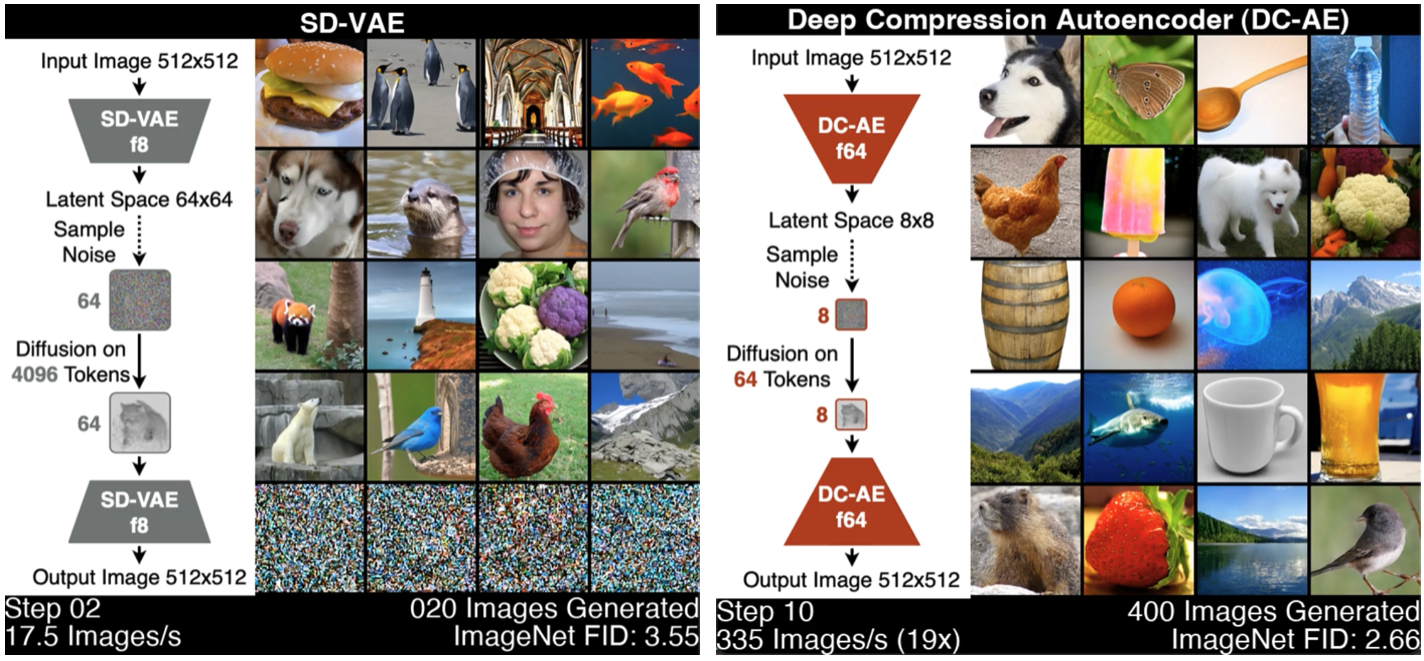
Deep Compression Autoencoder for Efficient High-Resolution Diffusion Models
DC-AE is a new autoencoder family for accelerating high-resolution image generation. DC-AE solved the reconstruction accuracy drop issue of high spatial-compression autoencoders, improving the spatial compression ratio up to 128 while maintaining the reconstruction quality. It dramatically reduces the token number of the latent space, delivering significant training and inference speedup for latent diffusion models without any performance drop.
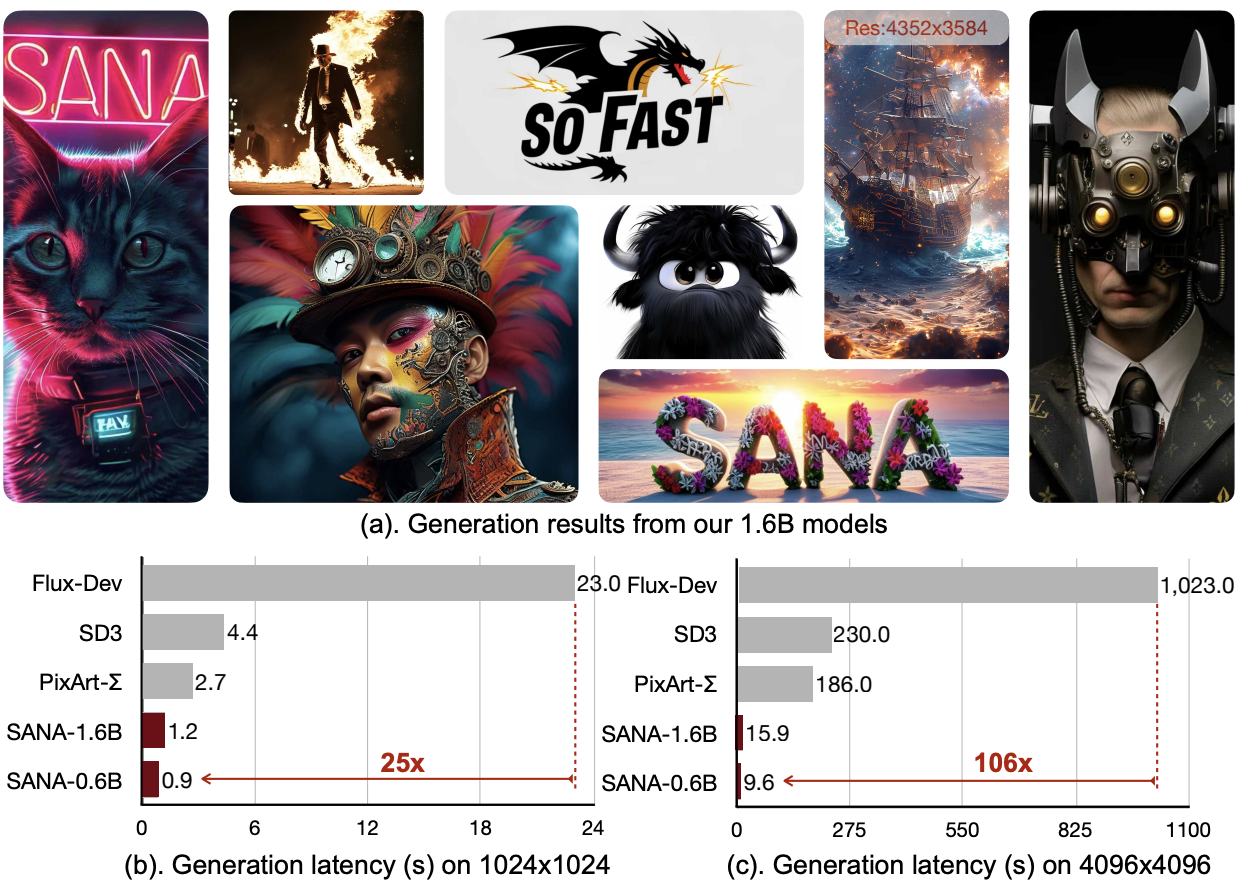
SANA: Efficient High-Resolution Image Synthesis with Linear Diffusion Transformers
SANA is a text-to-image framework that can efficiently generate images up to 4096×4096 resolution. Sana can synthesize high-resolution, high-quality images with strong text-image alignment at a remarkably fast speed, deployable on laptop GPU. Core designs include: (1) Deep Compression Autoencoder (2) Linear DiT: we replace all vanilla attention in DiT with linear attention, which is more efficient at high resolutions without sacrificing quality. (3) Decoder-only text encoder: we replaced T5 with decoder-only small LLM as the text encoder and designed complex human instruction with in-context learning to enhance the image-text alignment. (4) Efficient training and sampling: we propose Flow-DPM-Solver to reduce sampling steps, with caption labeling and selection to accelerate convergence.
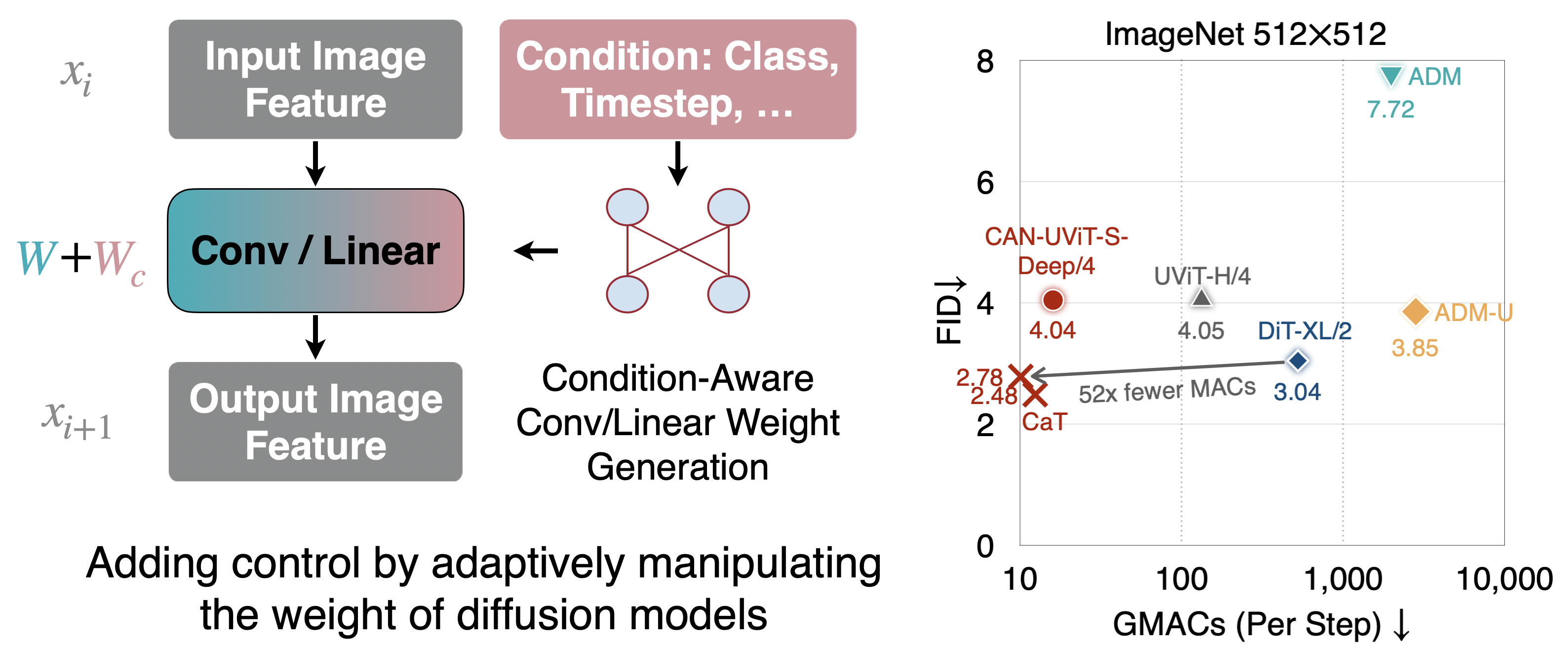
Condition-Aware Neural Network for Controlled Image Generation
CAN is a new method for adding control to image generative models. In parallel to prior conditional control methods, CAN controls the image generation process by dynamically manipulating the weight of the neural network. CAN combined with EfficientViT (CaT) achieves 2.78 FID on ImageNet 512x512, surpassing DiT-XL/2 while requiring 52x fewer MACs per sampling step.
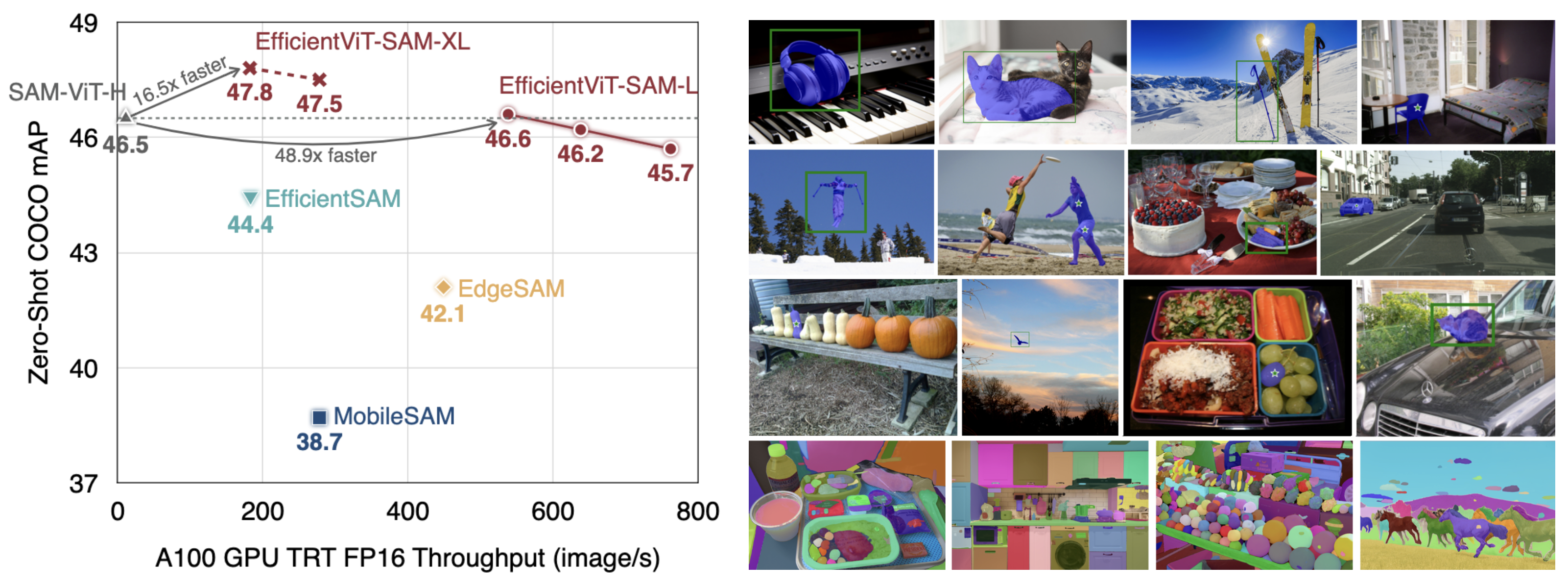
EfficientViT-SAM: Accelerated Segment Anything Model Without Performance Loss
EfficientViT-SAM is a new family of accelerated segment anything models. We replace SAM's heavy image encoder with EfficientViT. Benefiting from EfficientViT's efficiency and capacity, EfficientViT-SAM delivers 48.9x measured TensorRT speedup on A100 GPU over SAM-ViT-H without sacrificing performance.
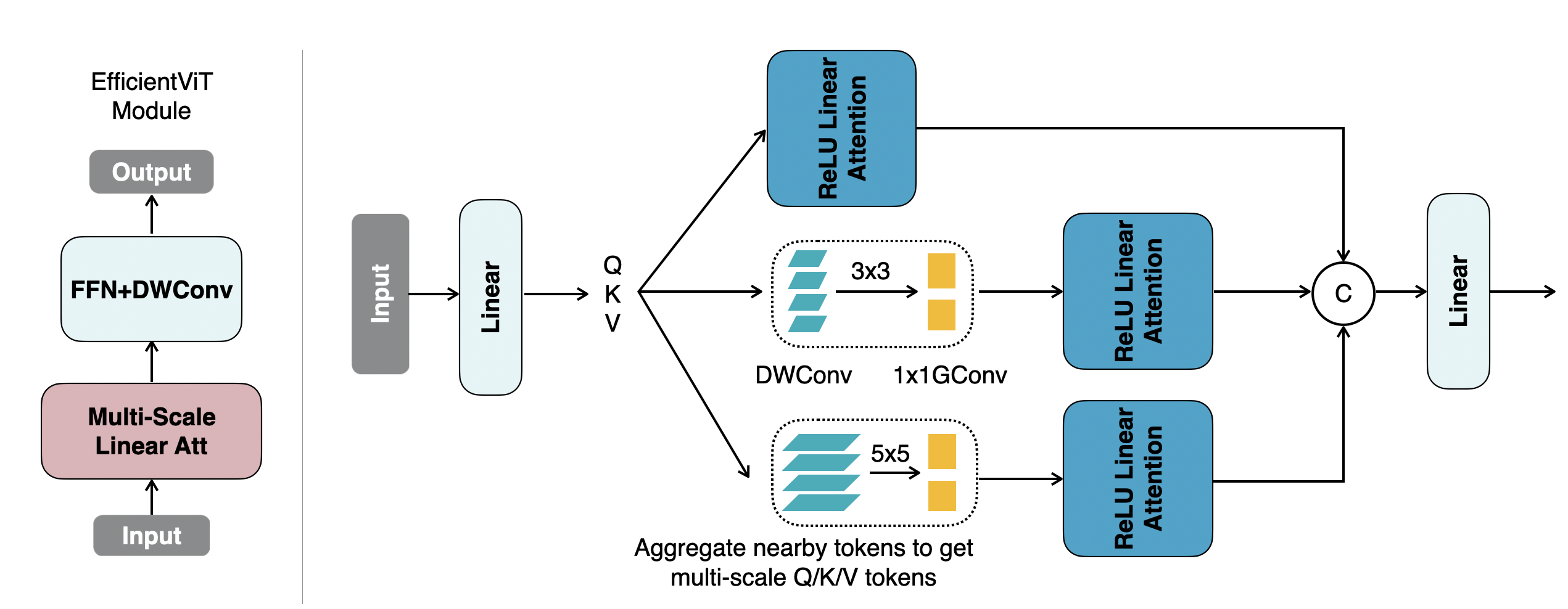
EfficientViT: Multi-Scale Linear Attention for High-Resolution Dense Prediction
EfficientViT is a new family of vision models for high-resolution dense prediction. It achieves global receptive field and multi-scale learning with only hardware-efficient operations. EfficientViT delivers remarkable performance gains over previous models with speedup on diverse hardware platforms.
[Media: MIT home page, MIT News, Imaging and Machine Vision Europe]
[Industry Integration: NVIDIA, HuggingFace]
[Code: GitHub (2.3k stars)]
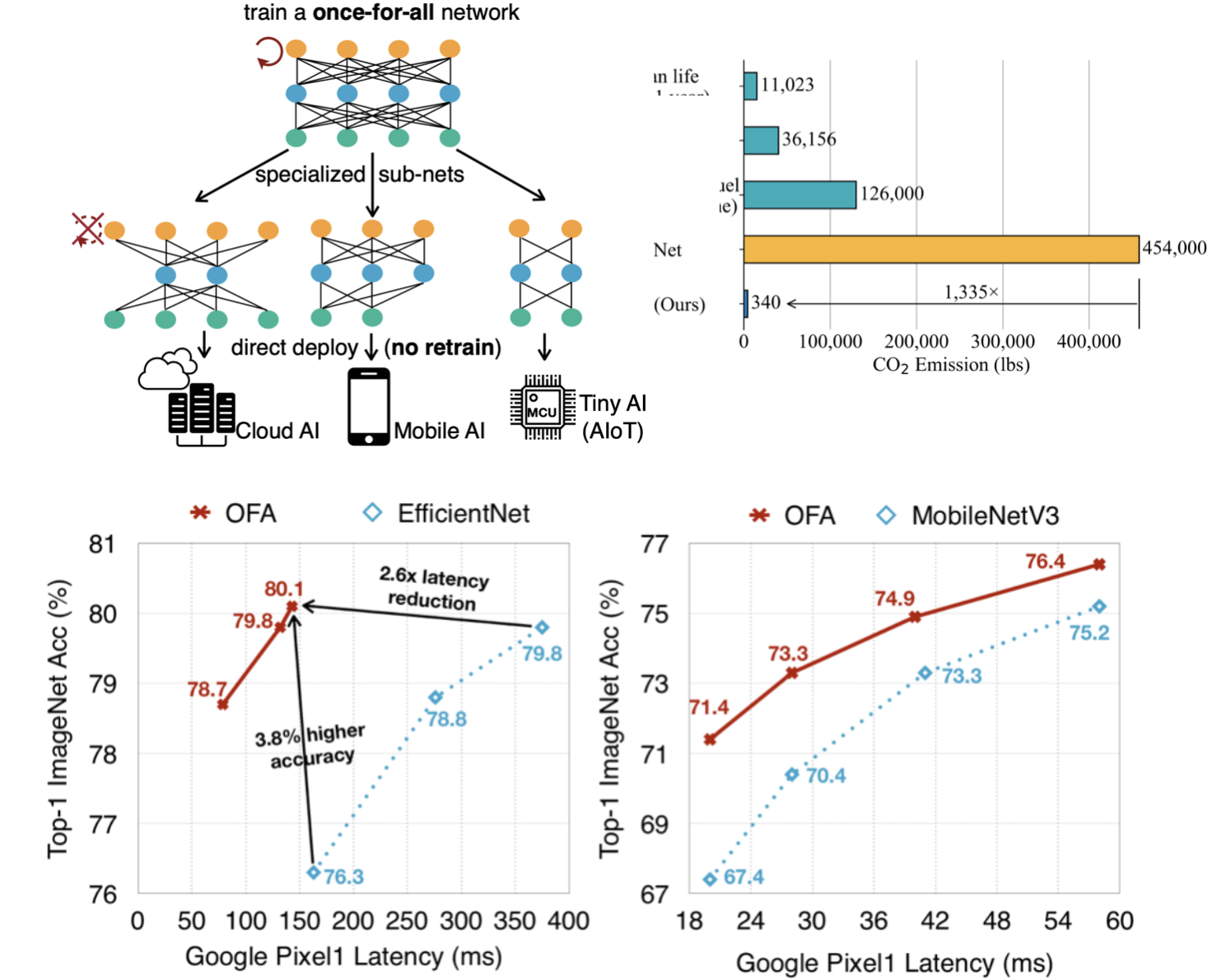
Once for All: Train One Network and Specialize it for Efficient Deployment
OFA is an efficient AutoML technique that decouples model training from architecture search. Train only once, specialize for many hardware platforms, from CPU/GPU to hardware accelerators. OFA consistently outperforms SOTA NAS methods while reducing orders of magnitude GPU hours and CO2 emission. In particular, OFA achieves a new SOTA 80.0% ImageNet top1 accuracy under the mobile setting (<600M FLOPs). OFA is the winning solution for CVPR 2020 Workshop of Low-Power Computer Vision Challenge (FPGA track), 2019 IEEE Low-Power Image Recognition Challenge (classification and detection track), Low-Power Computer Vision Workshop at ICCV 2019 (DSP track).
[Media: MIT News, Qualcomm, VentureBeat, SingularityHub]
[Industry Integration: Meta, Sony, AMD]
[Code: GitHub (1.9k stars), Colab]
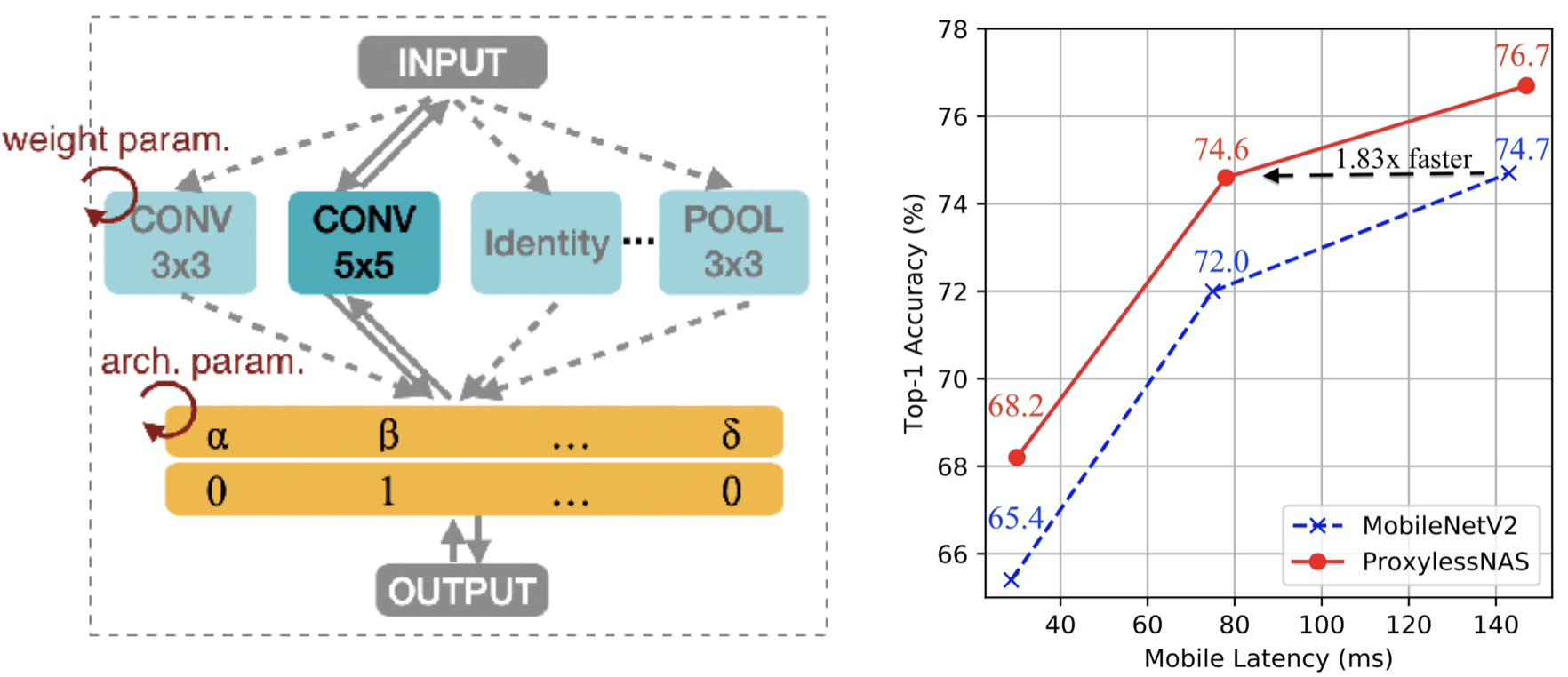
ProxylessNAS: Direct Neural Architecture Search on Target Task and Hardware
ProxylessNAS is an efficient hardware-aware neural architecture search method, which can directly search on large-scale datasets. It can design specialized neural network architecture for different hardware platforms. With >74.5% top-1 accuracy, the latency of ProxylessNAS is 1.8x faster than MobileNetV2.
[Media: MIT News, IEEE Spectrum]
[Industry Integration: Meta, Amazon, Microsoft]
[Code: GitHub (1.4k stars)]
Academic Services
- Serve as a reviewer for ICML, NeurIPS, ICLR, CVPR, ICCV, ECCV, TPAMI, IJCV, ACL, EMNLP